Being a Carbon Aware Developer
Understanding and mitigating the carbon footprint of AI development is increasingly important.
Future AI advancement is bottlenecked by its insatiable energy demand, a reality brought into sharp focus earlier this year when Microsoft and OpenAI unveiled their ambitious plans for a $100 billion AI supercomputer named Stargate. Projections indicate it would require an unprecedented amount of nuclear energy, the specifics of which remain unclear and untested.
Given this escalating energy demand, I’m motivated to become more carbon aware and energy efficient in my AI development. The "Carbon Aware Computing for Generative AI Developers" course offered by deeplearning.ai, in partnership with Google Cloud, provided valuable insights into the carbon emissions produced throughout the machine learning (ML) model lifecycle, outlining the main factors to consider:
Hardware: Everything from GPUs, TPUs, and servers to RAM contributes to what’s called "embodied carbon". The complexity of global supply chains makes it difficult to accurately calculate the total emissions across Scope 1, 2 and 3 levels associated with the production and shipping of these components.
Models: A wealth of specialized, smaller models offer greater energy efficiency. The Hugging Face LLM-Perf and ML.Energy leaderboards are valuable resources for comparing energy costs alongside a model’s performance metrics.
Data Centre Location + Time of Day: Where and when you train models greatly impacts carbon emissions - this is measured by the grid’s carbon intensity (gCO2eq/kWh), which represents the carbon emissions per kilowatt-hour of electricity and is determined by the local energy mix. Low-carbon energy sources like wind, solar, hydro, and nuclear are ideal.
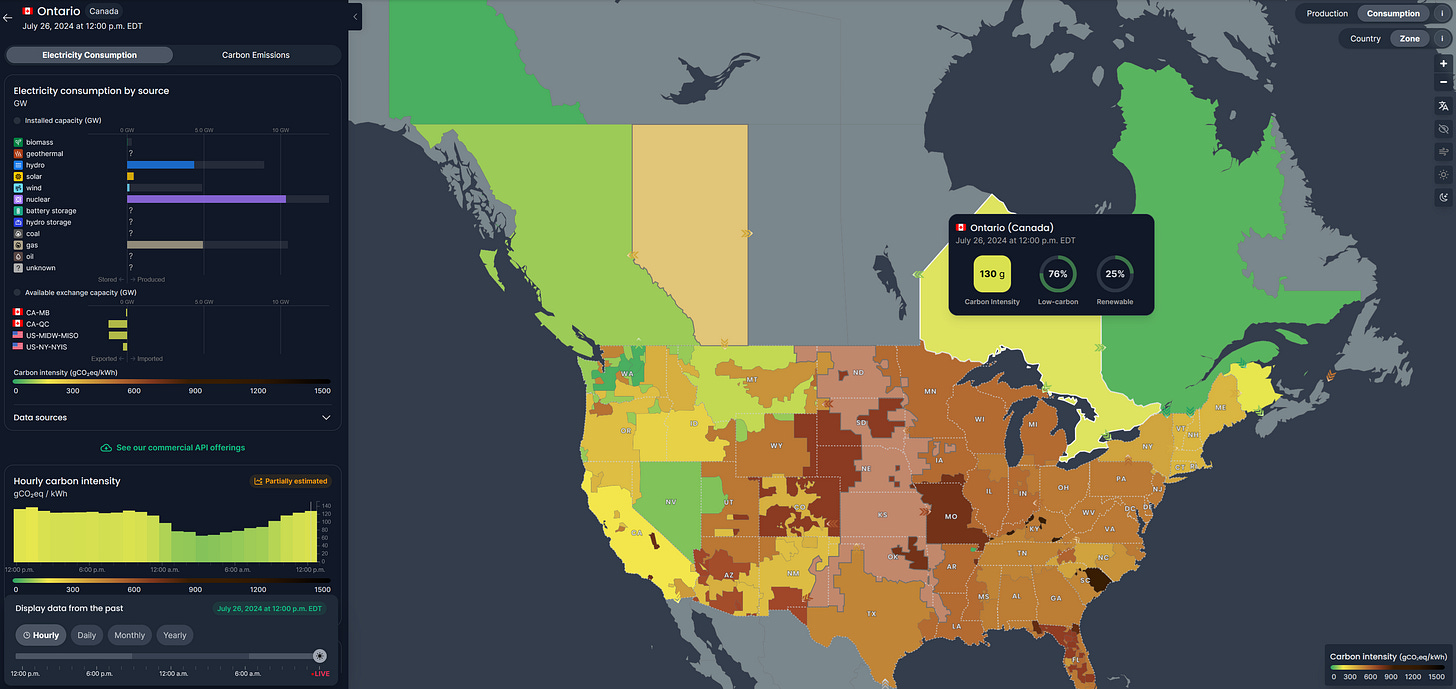
Google is helping the industry adopt dynamic strategies like shifting computing workloads to different servers throughout the day to "follow the sun and wind," taking advantage of the times and locations where low-carbon energy is most abundant. This approach is part of their commitment to ‘boldly accelerating climate action with AI’, but their overall carbon footprint remains a significant challenge.
As well, Google Cloud's region picker offers an overview of the different grid carbon intensities for each of its 40 data centres around the world, using data from Electricity Maps.
Focusing on Inference: The Carbon Cost of Thinking
A recent paper, "Power Hungry Processing: Watts Driving the Cost of AI Deployment?", by developers at Hugging Face and Carnegie Mellon University reveals a wide variation in the carbon footprint of AI model "inference" - the process of using a trained model to make predictions or generate responses. This ongoing energy use is comparatively harder to measure than the significant, one-time energy cost of training.
The researchers tested 88 different models across 30 datasets and 10 task types, highlighting the stark differences in their computational and energy demands:
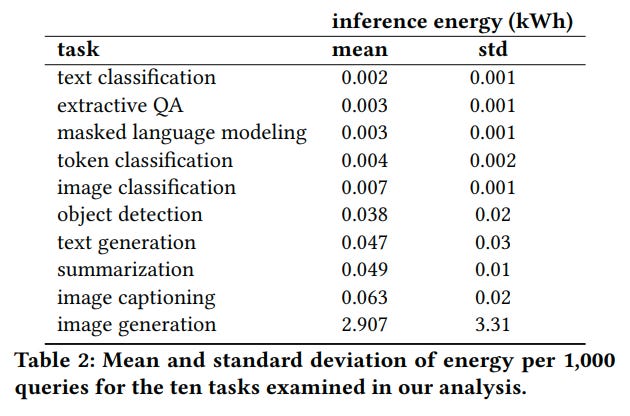
The authors emphasize that using “multi-purpose” models (i.e., LLMs) for discriminative tasks like classification is far more carbon-intensive than using task-specific models. While convenient for users and easy to deploy, their widespread use in digital products like web search and email can often be overkill, contributing to a substantial and unnecessary carbon footprint. Developers can significantly reduce energy consumption by using smaller, more specialized models when appropriate — from 3x less for content generation to 30x less for discriminative tasks.
Sasha Luccioni, one of the paper's authors and a member of the Hugging Face team, is advocating for Energy Star Ratings for AI models. Inspired by the familiar ratings system for appliances and devices, this would establish a standardized methodology for quantifying energy consumption and carbon emissions of ML models.
Prioritizing energy efficiency in AI development and incorporating carbon emissions as an essential metric in conjunction with performance, speed, and security is pragmatic, and I look forward to the industry adopting more sustainable practices and innovating in this critical area.