Geospatial AI: Seeing Earth Anew
Discovering how AI is evolving geospatial analysis, illuminating the dynamics of our rapidly changing planet through satellite imagery.
In my operations roles at startups, I've found mapping tools to be quite powerful for visualizing data and extracting insights to inform real-world business outcomes.
Expanding my perspective to encompass urban planning and environmental sustainability, I'm inspired by the power of geospatial analysis and the advanced, rich visualizations that better describe the interconnected challenges and opportunities facing our communities and our planet.
Since the development of Geographic Information Systems (GIS) in 1969, remote sensing technologies like satellites have revolutionized our ability to observe and understand Earth, providing crucial data for confronting the urgent challenge of climate change.
Foundational Satellite Programs
Programs like ESA Sentinel-2, NASA Landsat, and NASA MODIS have been instrumental in offering open public data for comprehensive Earth observation for decades. This data covers most of the globe, enabling researchers, decision-makers, and the general public to access valuable insights about Earth.
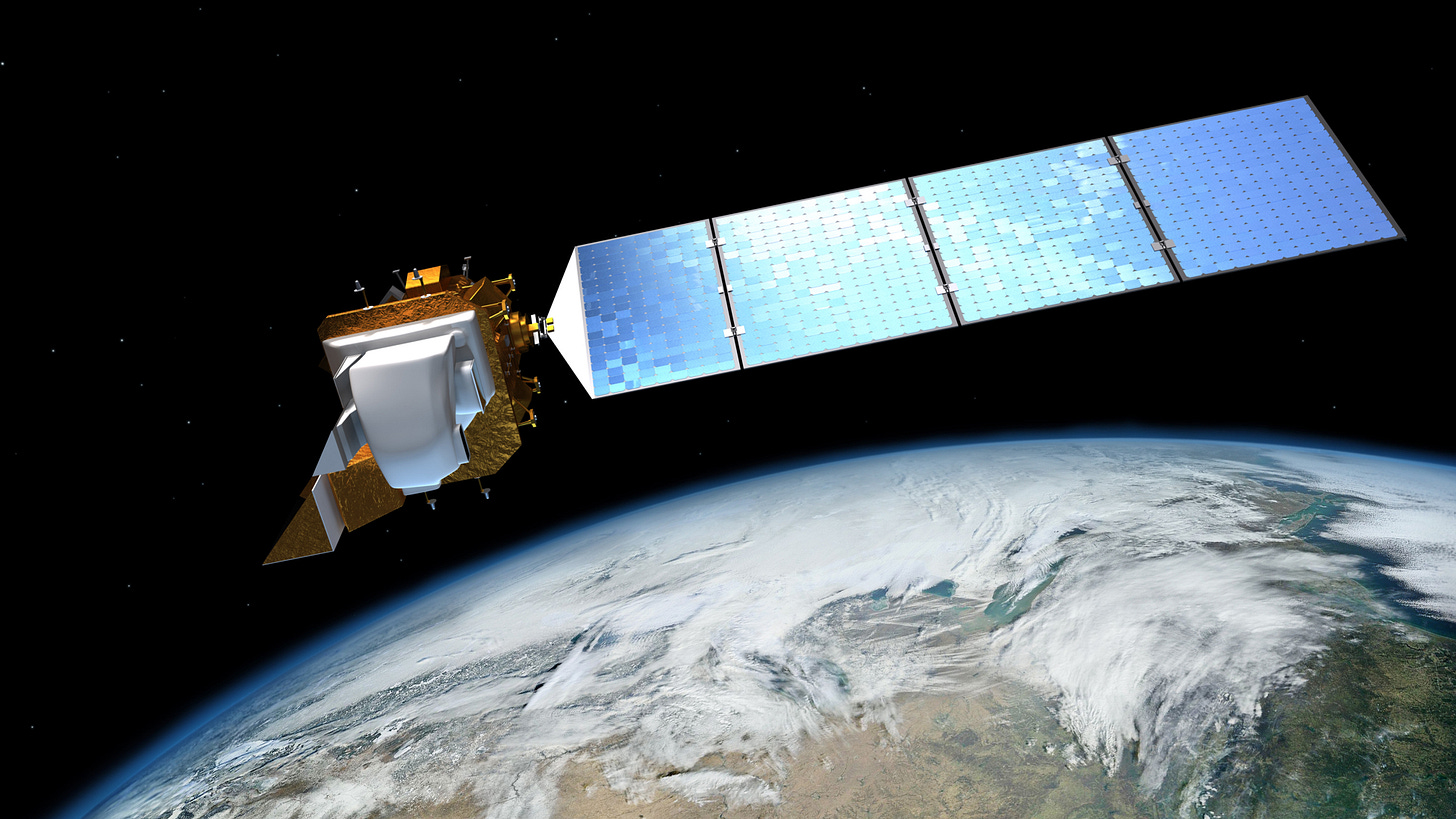
Satellite imagery is characterized by three key dimensions:
Spatial: The location of objects on Earth's surface using latitude, longitude, and altitude. The spatial resolution, typically measured in metres per pixel, determines the level of detail captured in the image. For example, Landsat 8 and Sentinel-2 have impressive resolution ranges from 10 to 60 metres. In contrast, commercial options can achieve even higher resolutions, down to 15 to 50 centimetres per pixel.
Spectral: Captures the unique interaction of materials with different wavelengths of light, whether reflected or emitted. By using multiple spectral bands beyond the visible spectrum, we can 'see' the Earth in novel ways, revealing detailed information about the composition and properties of different surface materials.
Temporal: Tracks how frequently a satellite revisits a specific point on Earth, allowing us to monitor changes over time, uncovering meaningful patterns in the progression of weather patterns, landscapes, and human activity. Sentinel-2 and Landsat 9 have a revisit time of about 5 and 8 days respectively, while MODIS, along with private, specialized satellites with wider swaths, can revisit most locations daily (and multiple times at that).
Embeddings for Geospatial Intelligence
AI is transforming how the geospatial industry processes, visualizes, and extracts predictive insights from satellite imagery. At the heart of this transformation are embeddings—mathematical representations of input data, like text, images and audio, that enable more efficient and context-aware processing by machine learning models.
This marks a paradigm shift from traditional pixel-based analysis, which focuses on individual pixels in isolation, towards a more holistic understanding of satellite imagery. Object-based image analysis (OBIA) was an important intermediate step in this direction, facilitating a grouping of pixels into meaningful objects that laid the foundation for understanding spatial context.
Embeddings further advance this approach, encoding data into hundreds or even thousands of dimensions to capture complex patterns and relationships far beyond the 2-3 dimensions that humans can intuitively grasp.
By leveraging the self-attention mechanism used in language models, AI models can understand context in Earth observation, just as they do in sentences:
Words: The word "bank" in “I’m going to the bank after work” refers to a financial institution, whereas in “It’s nice to hang out by the river bank,” it describes the side of a river.
Satellite Image: A tree in a city park might be recognized as part of an urban green space, while the same tree in a dense forest is interpreted as a component of a natural ecosystem. Improved classification through embeddings allows us to more accurately assess the rich dynamics of our built and natural environments.
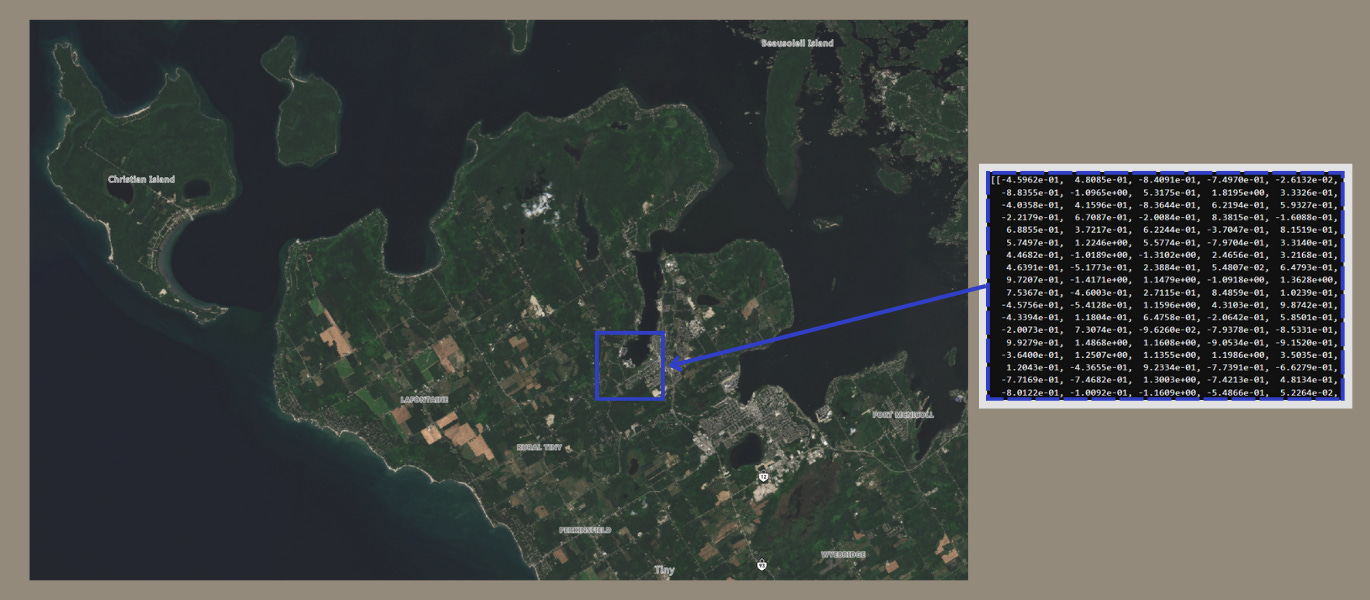
Advancing Earth Observation
The contextual analysis of Earth observation data by AI models enables more accurate and faster object detection, change detection, land cover classification, and crop yield estimation.
In turn, enhanced geospatial analysis powered by open-source tools and data deepens our understanding of Earth and opens up exciting new possibilities for data-informed, sustainable solutions.
Clay, an emerging non-profit open-source project, is significantly contributing to this evolution. Their AI model provides a robust foundation for advanced satellite imagery analysis and integrates it with other data sources. Through their AI for Earth Challenge, they encourage further customization and fine-tuning for targeted applications.
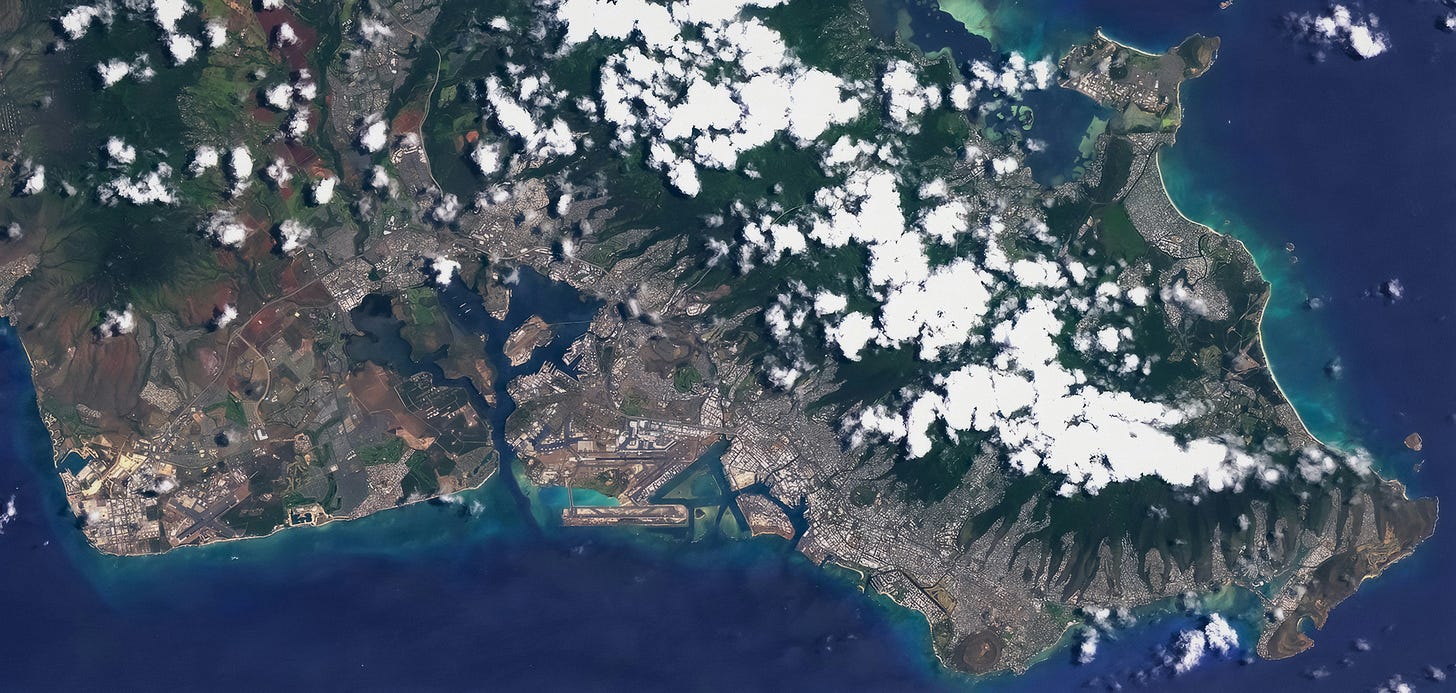
Clay’s Executive Director envisions a future where Earth observation is as accessible as a Google search—imagine searching for "muddy waters", "flood prone areas", or "docked container ships" just as easily as we find restaurants or hotels today.
Discover Your World
The open availability of satellite geospatial data makes this vision both achievable and inclusive, inviting us all to explore our planet like never before.
Platforms such as NASA Worldview, Sentinel Hub EO Browser, and Microsoft's Planetary Computer, among others, offer essential tools for accessing and analyzing this data, supporting a wide range of innovative applications and research.