Open Source AI: An Internet of Models
A close look at the rise of open-source AI models + opportunities and challenges for a more accessible and sustainable technological landscape.
Open-source software has been a driving force in technology's progress since the early days of the Internet. By making code publicly available, it fosters collaboration, transparency, and innovation among researchers, developers, and innovators worldwide, ultimately leading to better and more accessible software.
Democratizing access to powerful tools has empowered users and companies with systems like Linux, Firefox, Wikipedia, and countless others. Open source components are found in virtually all industry codebases, improving time-to-market, cost savings, and development resources.
Models: The Building Blocks of LLMs
The history of machine learning (ML) and natural language processing (NLP) is built on increasingly sophisticated models, from early text and voice recognition to image recognition and recommendation systems. In 2021, Stanford researchers coined the term “foundation models” to describe a new category of models trained on broad data and adaptable to a wide range of tasks. This laid the groundwork for the Large Language Models (LLMs) that have rapidly emerged and become commonplace today.
The Center for Research on Foundation Models (CRFM) maintains a comprehensive leaderboard of top-performing models, offering a detailed overview of standardized benchmarks across various domains:
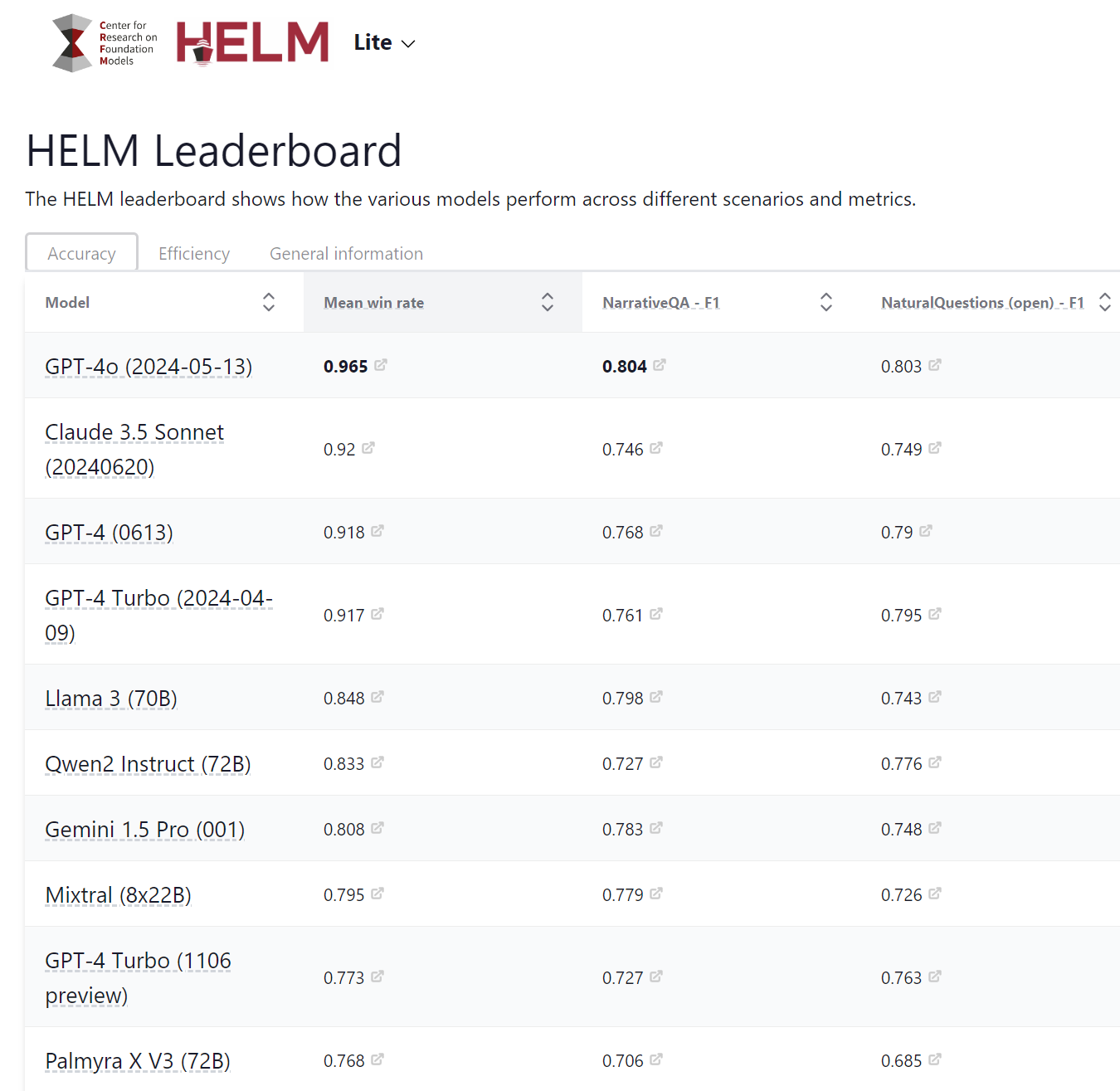
Advantages of Open-Source Foundation Models
While closed-source models, particularly those at the frontier of AI research, will likely always occupy the top spots on these leaderboards, the open-source LLM landscape is quickly growing, offering numerous benefits:
Enhanced customization and flexibility: A wider range of model sizes, indicated by their parameter count in billions (ex. 7B, 70B), allows for fine-tuning to specific needs and computational resources, ensuring greater accuracy and adaptability, especially for niche applications.
More efficient computational costs: Often have lower computational requirements and provide a superior performance-to-cost ratio, as demonstrated by the CRFM leaderboard for 'Efficiency.' This efficiency translates to lower energy consumption and a smaller carbon footprint, making them more sustainable.
Transparency: The ability to assess an LLM's training data and architecture allows for easier bias detection and mitigation, ensuring more accurate and fair outcomes. This openness fosters scrutiny and improvement of the technology, building trust and accountability.
Platforms like Hugging Face, a popular open-source AI platform, serve as an invaluable hub for the vibrant machine learning ecosystem by hosting hundreds of thousands of models (over 761,000 at the time of writing), datasets, and applications. Leveraging these resources and experimenting with effective prompting techniques has been instrumental in my AI journey.
Building with Llama 3
Meta's Llama model series has quickly become one of the most popular open-source language models, with Llama 3 released earlier this year and already demonstrating performance comparable to commercial top benchmarked performers. Available in 8B and 70B sizes, I used the 70B model hosted by NVIDIA for my project for the Generative AI Agents Developer Contest by NVIDIA and Langchain.
Inspired to streamline my blog writing process, I developed an application that creates high-quality blog drafts using a specialized team of five agents. Each agent has a unique role, taking a series of inputs and iteratively refining a draft up to 5 times. They’re equipped with web search tools to gather relevant information and integrate it into the draft, showcasing the potential of basic AI agent systems for creative content creation. Check out this 1-minute demo video to see it in action:
From Open Source to Open
A larger 400B parameter version of Llama 3 is currently in training and slated for release later this year, promising state-of-the-art language capabilities, multimodality, multilingual support, and longer context windows (memory). However, with development costs estimated at about $10 billion, it's unclear whether this version will be released under the same community license agreement for research and commercial use. In a recent interview, Mark Zuckerberg's response when asked about open-sourcing such an expensive model was pragmatic: "As long as it's helping us then yeah."
It’s worth noting that Llama models are not truly open-source, but rather openly available with certain restrictions. A definition for open source AI is in development, but would at least entail sharing the source pretraining data and the full disclosure of its underlying architecture — something that Meta and large tech companies are unlikely to do. Although these open models can serve as strategic tools for large players to establish market dominance through “commoditizing their complements," their contributions are undeniably accelerating and democratizing AI adoption. This trend is evident in the open models offered by Microsoft, Google, and Apple.
An example of a truly open-source AI is OLMo from The Allen Institute for AI, a non-profit research institute, with an LLM framework designed to provide access to data, training code, models and evaluation code to empower academics and researchers.
How Open Will The Future Be?
The rise of open-source AI also confronts existing challenges, as highlighted in the 2024 Open Source Security and Risk Analysis Report. It stresses the need to improve open-source management, and highlights the importance of keeping components up-to-date to minimize high-risk vulnerabilities and protect against data breaches and malicious attacks.
For example, hackers have been abusing open-source code libraries by mimicking the names of legitimate Python packages. Awareness and due diligence is needed at all levels - from developers to government agencies, efforts in policy-making and regulation must prioritize these emerging challenges in open-source AI security.
As AI continues to evolve, we can expect a more decentralized landscape beyond cloud-based LLMs, with a diversity of models offering various capabilities and functions that are more energy-efficient and cost-effective. Open-source language models are poised to play a significant role in everyday devices and appliances, powering voice assistants, translation tools, and other utilities, ultimately making AI more accessible and affordable for everyone. This internet of models will be a critical component of responsible innovation, along with the decarbonization of AI.